Embrace perpetual beta
Acceleration is now constant. Infogain designs and builds intelligent platforms that unlock meaningful experiences, enabling authentic interactions between you and your customers.
See What We DoEngineering Business Outcomes
We enable meaningful, measurable impact—and deliver business outcomes that create the future you want.
Innovate for Growth
Honor your past and move forward confidently. Our Fortune 50 travel customer grew annual revenues from $50 million to over $3 billion.
Read the Success Story Read the Success Story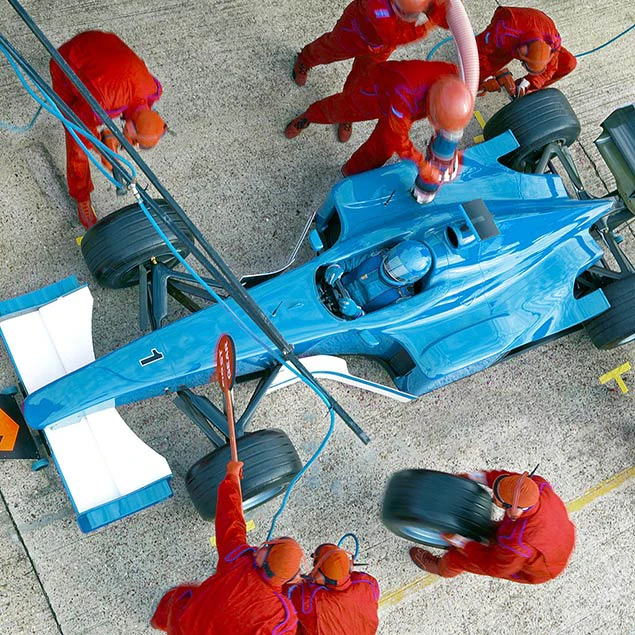
Design for Experience
The right experiences drive loyalty. Our Global 50 electronics customer integrated digital and physical customer experiences with AR and VR.
Read the Success Story Read the Success Story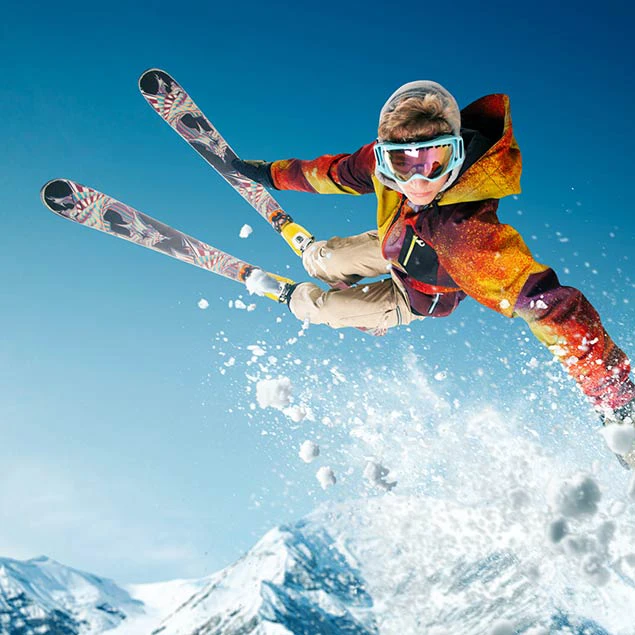
Engineer for Reliability
Reliable experiences delight customers. Our multi-billion-dollar travel customer automated testing to improve productivity and stability.
Read the Success Story Read the Success Story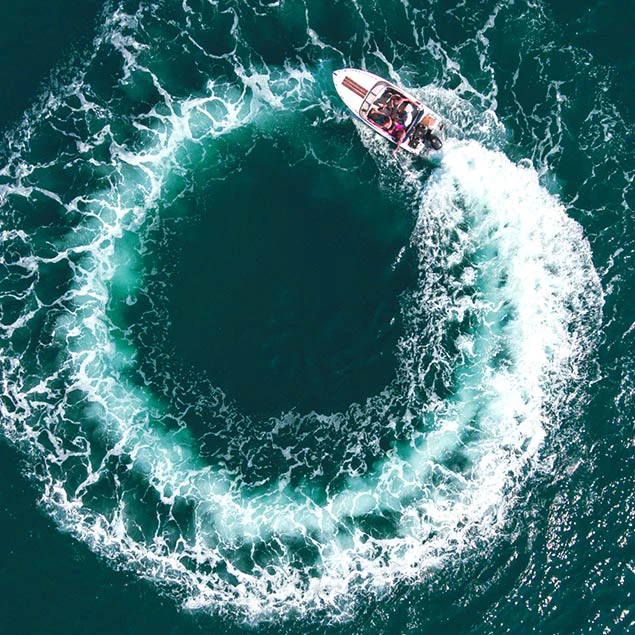
Accelerate with AI
Solve the unsolvable. Our multi-billion-dollar insurance customer moved to Agile and automated their DevSecOps pipeline.
Read the Success Story Read the Success Story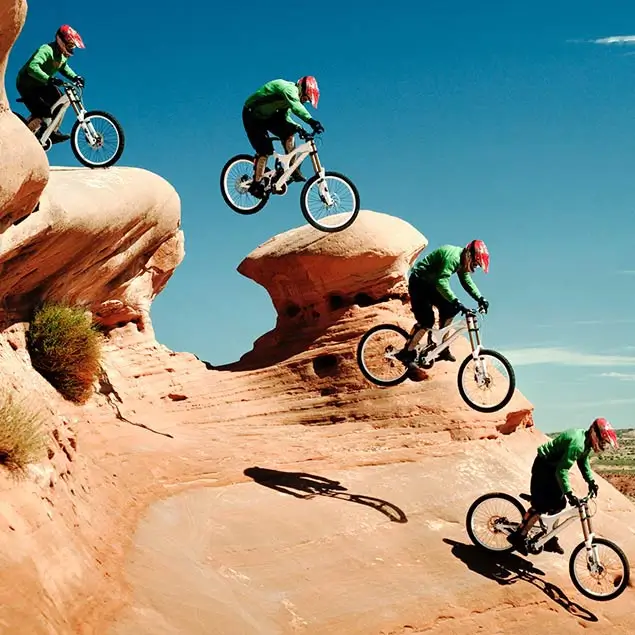
Release with Speed
Add to the bottom line now. Our online property listing customer used Google AI to lower costs and double activation rates.
Read the Success Story Read the Success Story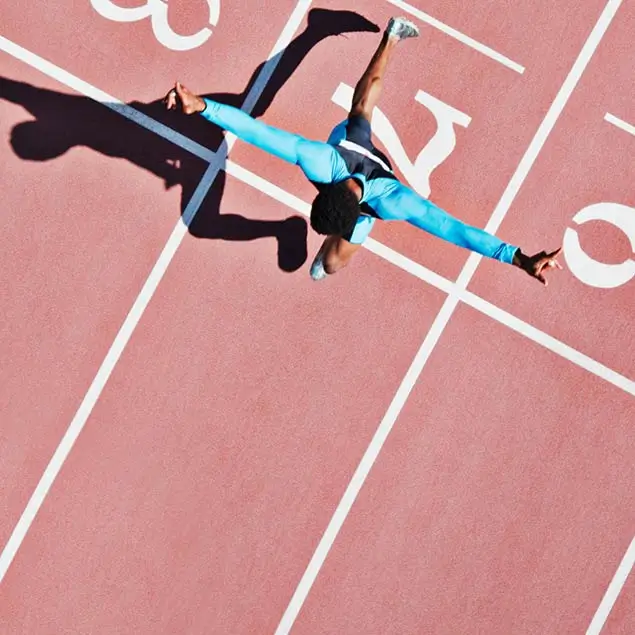
Wise answers to smart AI questions
We’ve been developing and adapting Generative AI long before it became a buzzword. We can help you get started today, realizing and maximizing the competitive advantage the technology — and our expertise — offers.
PROFIT FROM OUR EXPERIENCE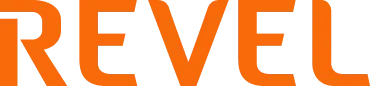
Focus on the human
Revel is the division of Infogain focused on Digital Innovation, Experience Design, and Transformation. Revel enables customers to navigate the unpredictable and redefine their platforms and products.
Explore RevelTransformation never stops
Users change faster than ever. The Infogain Value Delivery Framework and NAVIK AI let you turn proven digital blueprints into responsive platforms, then use AI-driven insights to capture customer needs, scale to serve employees and customers, and launch feature after feature in extensible ecosystems.
Move ForwardClient Speak
“…between the Coalfire Nation and the Infogainers, … we’re all trying to solve a common problem, which is helping organizations move, become more digital, become more software enabled and identify and solve some of the hardest challenges.”
– Tom McAndrew, CEO – Coalfire
Move your career forward
Contribute, collaborate, and lead with Infogain. Check out our open positions in the US, India, UK, Singapore, UAE, and Poland.
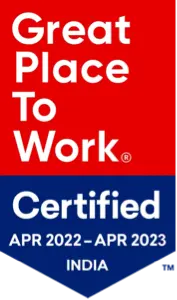
See who’s changed their own future
We've got decades-long relationships with the biggest brands in the world.
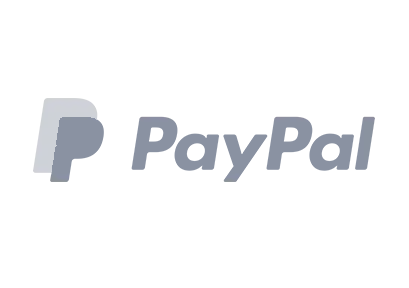
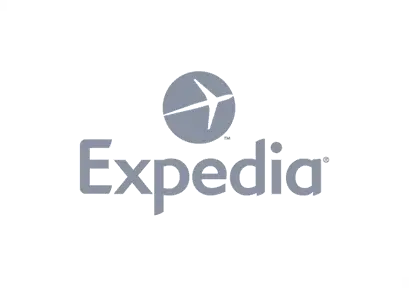
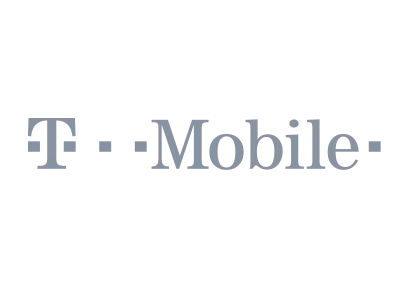
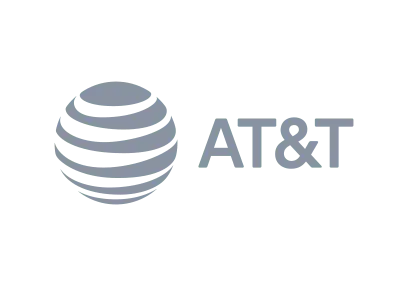
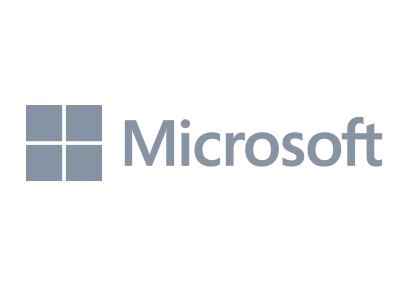
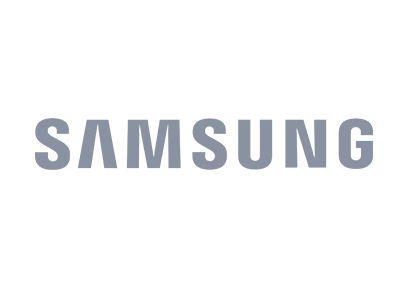
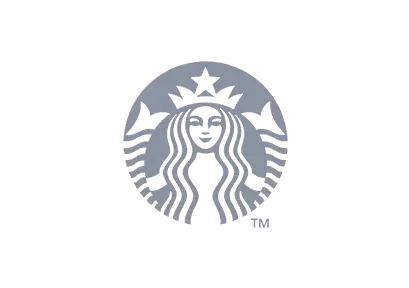
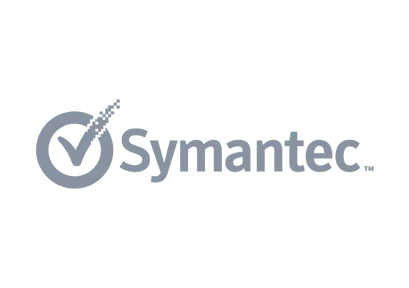
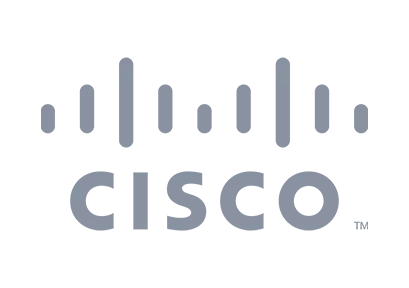
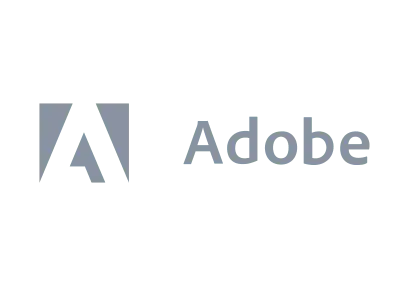
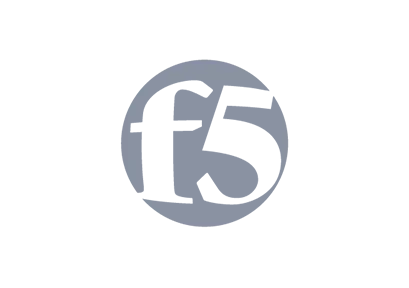
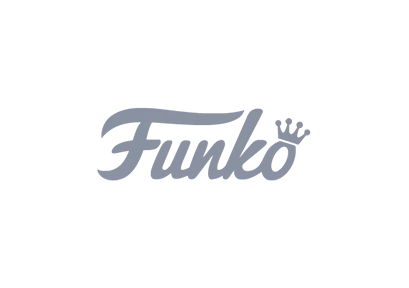
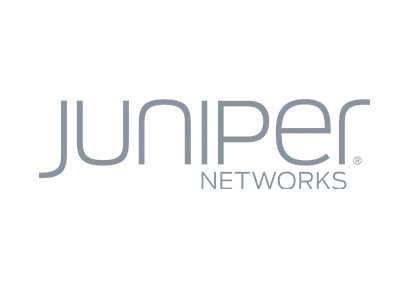
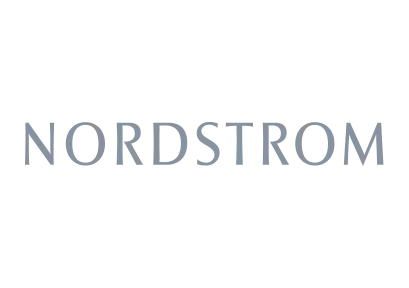
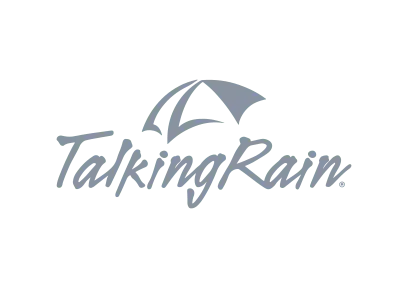
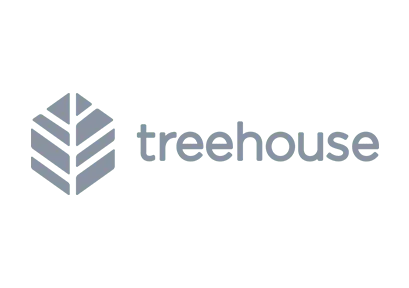
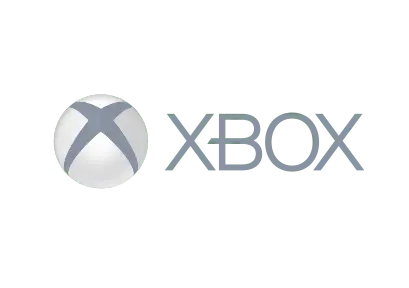