- Posted on : April 21, 2022
-
- Industry : Corporate
- Type: Blog
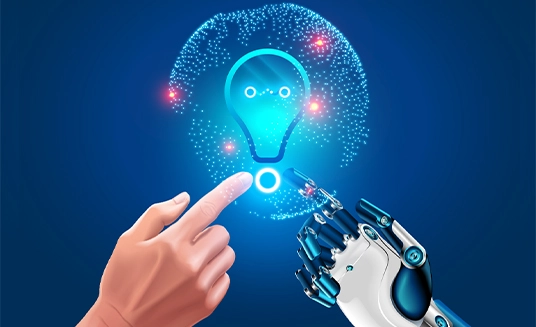
First there was data. Soon afterward came data analysis and then data analytics, Big Data, AI, machine learning, predictive analytics, natural language processing … The list of ‘Big Things’ in the business analytics world seems to get a new addition every year. Will 2022 be the year of the digital twin?
What is a digital twin, you ask? It’s a computer model of a real entity; there have been digital twins made to match supermarkets, human organs, public utility systems, and entire cities. As such, digital twins can be used to proactively monitor the current state of an object (such as a traffic grid) and to run various simulations, from simple what-ifs to complex predictive scenarios and routines.
Digital Twins vs. Predictive Analytics
Does some of this sound a bit familiar? Don’t we already have predictive analytics to run scenarios against? Yes, of course. So what’s the difference between digital twins and predictive analytics?
There are two subtly different answers to this question.
One is that predictive analytics uses digital twins. Predictive analytics is an umbrella term for running real-world data against artificial intelligence systems to determine what might happen under different future scenarios. And a digital twin is a type of model, albeit a complex one; it can certainly be used in predictive analytics.
The second answer refers to what business analytics users might mean by the term ‘predictive analytics.’ In this context, they might have in mind a simpler model that runs a test against limited variables (e.g. how price changes might affect product sales). In this case, we can say that a digital twin is a more precise and complicated version of a predictive model. Instead of using stored data, it uses dynamic, real-world data streams. Instead of testing for just a few variables, it can take many variables into account. Instead of looking at just a handful of outcomes in one precise area, it can generate a picture of the whole.
Under this definition, you could use the illustration that a predictive model is like examining one part of the production line, while a digital twin is like monitoring the status of the entire production facility.
A key difference between digital twins and other computer models is the quality and timeliness of incoming data; digital twins have a real-time connection to the thing they represent. For example, the digital twin of a computer manufacturing plant would get real-time data from sensors built into the production machines; a utility grid would get real-time usage data, and so on.
An article in SAP Insights takes the interconnectivity of digital twins a bit further, noting that in the future, “digital twins will form their own networks, which experts call a “digital thread.” If a digital twin enables us to create a digital representation of a piece of equipment or facility, then a digital thread is the continuous, connected stream of information provided by an intelligent asset throughout its life cycle.”
Why Digital Twins Are Generating Buzz Right Now
Digital twins have been around for decades, and they’ve been hovering in the business collective consciousness for several years now. Why has the buzz been getting louder of late?
One reason is that computing technology has been evolving enough to support the widespread use of digital twins; it’s no longer the sole domain of well-funded research departments. Similarly, AI and data analysis have become more affordable and available. Thus, digital twins are more affordable as well. Another plus is that more enterprise tools and platforms can be integrated with digital twin capabilities.
As with so many other things in business tech, digital twins’ surge in popularity is a combination of cost, availability, technical growth, and (of course) the massive amount of data we can now access.
Another reason is that companies – having seen the benefits of AI and data analytics – have now begun to recognize the possibilities of digital twins. Currently, we deploy various AI and machine learning models to solve specific problems; with a digital twin, we could provide business insights for an entire organization. We can use it to model buying behavior with exactness, to build a better customer experience, to forecast consumer demand. We can do all of those things using current AI tools, but with digital twins, we can do them better and faster.
Other interesting possibilities open up when we think of applying digital twins to areas like customer service. For example, a digital twin might help e-commerce stores analyze usage patterns and adapt their apps or websites according to the personal preferences of each customer. Or they could help minimize clients’ frustration in the customer service process, both proactively (testing out improvements before they are offered to the public) and reactively (analyzing the current process and isolating the causes of bottlenecks and pain points).
A Sharper Vision with Digital Twins
Another way to look at the advantages of digital twins is to imagine how we view organizations. Right now, we’re looking at a composite picture that’s stitched together from several smaller (department or business area) pictures. We have a good likeness of our organization, but the pictures are a bit grainy and the joins are evident. With digital twins, we could have a live panoramic view of what’s going on in real time. Remember, a digital twin is a model of a real-world object, and as such it’s continually being updated. As companies lean ever harder into becoming data-driven, digital twins look set to be the next wave of business AI.