- Posted on : October 10, 2017
-
- Industry : Digital and High Technology
- Type: News
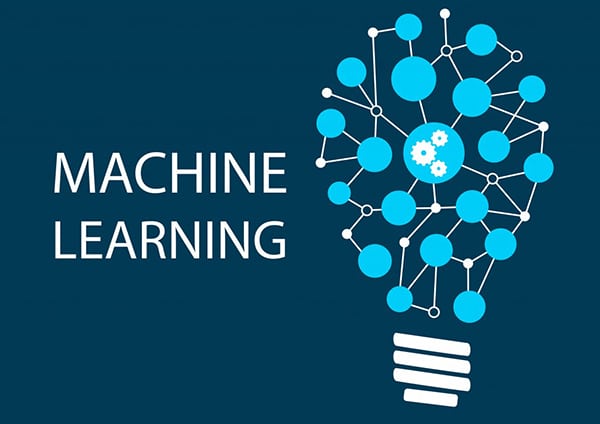
Contributed By Ramesh Subramanian, CTO, Infogain
Machine Learning—a subset of Artificial Intelligence(AI) is the latest buzzword in the technology industry which is emerging as the pathway to the future for enterprises. Machine Learning provides enterprises with the required framework, insights, and algorithms to ensure better predictive ability.
The ever-increasing usage of electronic means of interaction and commerce, as well as IoT devices has been producing an incredible volume of data and statistics which is impossible for humans to analyse manually. ML technology helps combine all the data gathered from myriad touch points for delivering useful insights to enterprises that contribute to the various strategic outcomes.
A survey conducted in 2016 by the National Business Research Institute revealed that 62 per cent enterprises will deploy AI technologies by the year 2018.It is evident that both Machine Learning and AI is becoming a vitalfacet for several burgeoning as well as eminent industries as it can offer deeper insights to businesses besides enhancing the process of decision-making.
Machine Learning allows computers to be capable of categorizing, processing, and generating data based on buying and spending patterns of customers, their feedback and interactions, their peers and social groups, and virtually anything else.
An ML-based computer algorithm can identify which customers are most likely to abandon your brand besides helping you profile their identity, buying habits, and the reasons that are making them leave your brand.While such use-cases for ML abound, enterprises are adopting ML algorithms to increase flexibility of shop floors, supply chains, collaborative partnerships, even detect the price points that consumers will prefer.
How are Enterprises Adopting Machine Learning?
More and more businesses are embracing this technology with open arms as it generates positive ROI and leads to the advancement of future products. Technology giant Google was among the first to recognise the significance of integrating ML technology; nevertheless, at the present time,deep-learning techniques have a wide appeal across industries. For example, financial institutions are using ML technology for identifying potential cases of fraudulent claims, and also for conducting a risk analysis. Similarly ML is helping healthcare providers save lives by identifying severe health issues by analysing patient data.
Today ML is controlling applications such as real-time speech translation, biometric identification system, gene mapping, web-content curation, and so on.In order to leverage Machine Learning enterprises are working in tandem with their customers to comprehend their grievances so as to make sure that their products and services address the specific needs of the customers.For example, Pinterest has been using ML technology to display more interesting content to you. Similarly, Disqus utilises ML to eliminate comment spams. Likewise, several e-commerce companies are employing ML strategies to provide their clients with the advantages of machine learning when the clients browse for products on their sites.
Enterprises that have incorporated ML technology into their business processes are mainly focusing on the following application areas—
- Text classification and text data mining,
- Natural language processing (NLP) that can generate reports by analysing texts and establishing connections between concepts,
- Image recognition, image classification, image tagging,
- Behaviour analysis and personalising customer service,
- Mapping and recommendations,
- Risk analysis and data security; &
- Smart Assistant like Microsoft’s Cortana and Apple’s SIRI.
Measurable Business Outcomes of Machine Learning
One of the crucial objectives of implementing ML technology in business is to transform business objectives into asses-sable goals.Here are some measurable business outcomes of machine learning—
- Helps enterprises in meeting measurable objectives by enhancing market share.
- Assists businesses in determining their success by analysing the number of new and existing customers which in turn helps businesses to assess its different marketing tactics.
- Enables businesses to measure the volume of feedback they receive from their customers or clients. This helps businesses to detect their weak points in their customer services besides allowing them to develop their overall service levels.
What kind of readiness is required for ML adoption?
In order to implement ML technology successfully, it is important for enterprises to ensure readiness across the following factors—
- Tactical Readiness: Enterprises should define their goals precisely for ML-based solutions. To begin with, they must identify the factors they are willing to measure. At the same time, they should identify the business problems they are trying to resolve with the help of analytics.
- Readiness for a Data-driven Culture: Most ML-based solutions need lots and lots of quality data.Enterprises should ensure that they are geared up for making data-driven decisions by building a strategic analytics culture. The company’s leadership should be willing to adopt the insights generated by the data analytics team and utilise the analytical outcome for decision-making.
- Domain Readiness: Enterprises should build an infrastructure that can access and evaluate data effortlessly. This can be done by investing in a skilled workforce and providing training to the existing employees. Companies should also invest in analytical and ML tools besides investing in recruiting workers that are skilled enough to utilise the data. Domain readiness also entails thorough comprehension of an organisation’s business and data landscape.
- Functional Readiness: Successfully implementing a ML solution can call for a lot of preparation, timing, proper budgeting, and well-defined goals. It is crucial for enterprises to gauge success and discover the vital performance metrics for assessing progress towards the predefined organisational objectives. Moreover, enterprises should chalk out a precise dissemination strategy so as to convey analytic insights to those who require them.
ML Technology– Helping Enterprises Turn Unstructured Data into Competitive Advantage
Respondents of a study conducted by MIT Technology Review Custom in association with Google Cloud revealed that the most important advantage of ML technology is that it enables implementersto achieve a competitive advantage. The study further states that 25 per cent of existing ML implementers believe that they have already accomplished that goal.76 per cent respondents stated that they are utilising ML for escalating sales growth.
Here are few organisational goals cited by the survey that ML adopters can achieve by implementing this technology—
- Improved insights and data analysis;
- Better understanding of customers;
- Faster assessment and more prompt insight;and
- Better internal efficiency.
What kind of initiatives is required in the adoption of Machine Learning?
- Emphasize on Data: In order to generate accurate results, the data supplied to machine-learning algorithms should be labelled, cleansed, and organised in a proper manner. In order to transform the massive volume of unstructured data generated on a regular basis, enterprises may opt for internal data labelling and data scrubbing or they may opt for a third-party service provider. Simultaneously, enterprises should improve their ML readiness by organising and amalgamating their scattered data sources into an integrated data warehousing platform.
- Embrace the Latest AI Tools: AI innovators are encouraging the implementation of AI technology across industries by open-sourcing machine-learning libraries and Application Programming Interface (API). For example, Google’s open-sourced library TensorFlow enables enterprises to have access to unconventional algorithms and optimised neural networks for quick deployment in an organisational setting.Enterprises can also reap benefits from cloud-based Machine Learning APIs that makes the process of computing in-house AI software simpler.
- Develop Narrow Expertise: Though it is quite tempting to make use of ML technology in each and every business process, such a policy may exhaust enterprise resources besides reducing the progressive effect of AI advancement. Hence, businesses should give precedence to concrete artificial intelligence solutions having the greatest potential of enhancing monetary value and customer happiness. Developing narrow proficiency in a single area will go a long way in helpingenterprises utilise resources on a specific task, thus, facilitating the progression of an advanced solution for one’s business.
In today’s digital landscape, it is indispensable for businesses to incorporate machine-driven approaches.Since ML technology is playing a key role in driving an era of innovation, businesses should adopt machine learning for gaining a competitive edge in their respective industries.
— The writer is VP, HR, Infogain
News Originally Posted on: PC Quest